White paper
Accelerate EV battery development
with AI-guided test
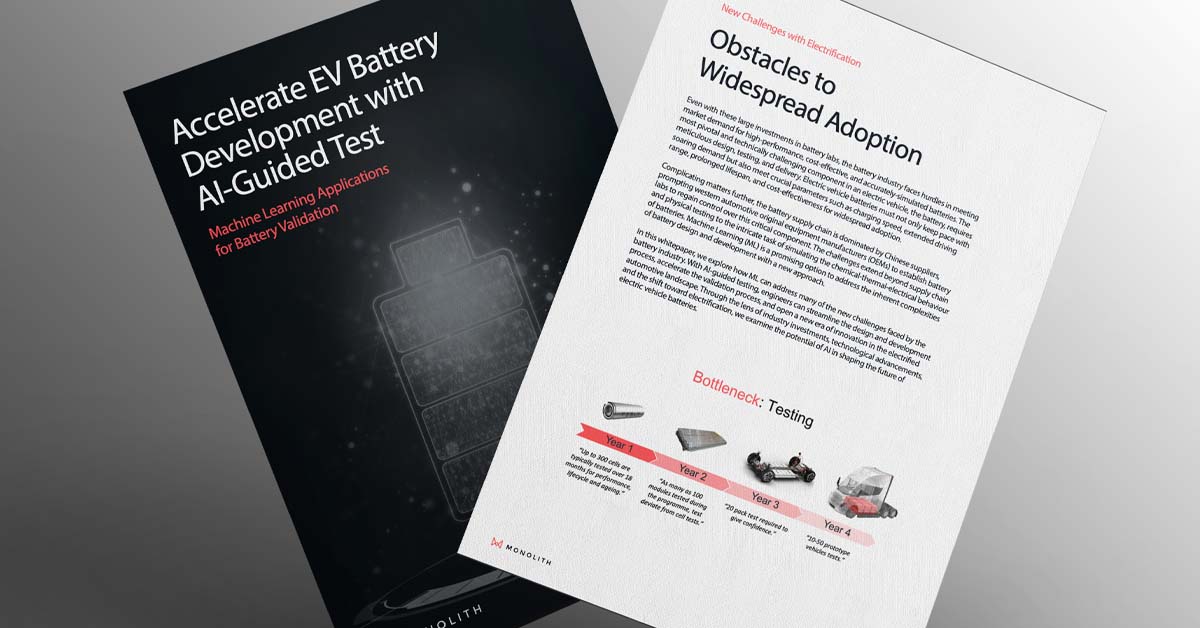
Executive summary
The automotive industry’s shift to electric powertrains brings new challenges for OEMs and suppliers. EV batteries introduce new technical, business, and supply-chain obstacles that must be overcome to drive widespread adoption. EV batteries are incredibly complex to design and simulate because of their challenging mix of electrical, chemical, and thermal characteristics. As a result, OEMs are investing massive amounts of time and money in the physical testing of batteries.
This document explores a different way of testing that uses artificial intelligence and machine learning. By training self-learning AI models from test data, engineering teams can speed up battery development and ensure batteries meet high standards for performance, reliability, and safety.
One main focus here is to present solutions beyond regular testing methods. Machine learning builds valuable insights into how batteries behave in different conditions, allowing engineers to reduce the time and effort spent on physical testing while increasing their confidence in how the battery will ultimately perform. By employing an AI-guided test strategy, test engineers can accelerate new products to market in the following ways:
• Build more efficient test plans that cover the most important battery test conditions in fewer steps
• Automatically inspect and identify test data that may be faulty when running complex, high-channel test systems
• Identify and address the root cause of system or test failures when they occur
Engineers can circumvent many of the challenges of traditional battery testing to get new products to market faster and with greater confidence, using AI-based, self-learning models.