Publication
Human-inspired generative design
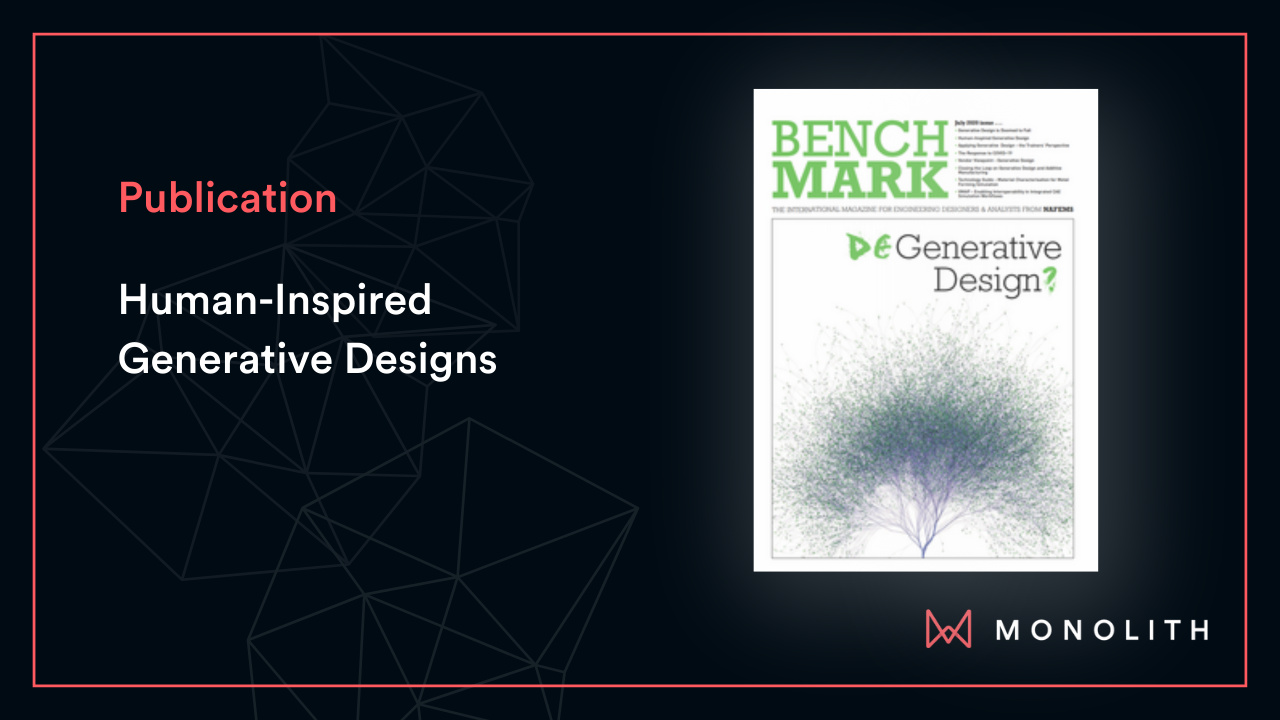
Abstract
Engineering is a highly creative discipline. Every iteration of simulations or tests provides engineers with new learning on how to best refine their design, based on complex goals and constraints. Finding an optimum solution means being creative about what designs to evaluate and how to evaluate them.
When trying to build an understanding of how a non-linear and multi-variable physical system works, all engineering efforts (simulations or physical tests) are journeys to learn functional relationships by analysing data. This data is based on ineradicable governing physical laws and relationships. Unlike financial data, for example, data generated by engineers reflects an underlying truth — that of physics, as first described by Newton, Bernoulli, Fourier or Laplace.
The fact that this data is abundant (generated from numerous design iterations) and based on fundamental scientific principles means that it can often be an excellent subject for a machine learning study: predicting the behaviour of a new design under new testing conditions, or optimising the design and conditions for a target behaviour.
Researching this possibility has been our focus for the last few years and we have, today, built numerous AI tools capable of considerably accelerating engineering design cycles.
In particular, our main focus has been to develop deep learning models to learn from 3D data such as CAD designs and simulations. The early adopters of our technology have found it to be a breakthrough. We strongly believe that the methods outlined in this article will revolutionise engineering in a similar way that AI for facial recognition has revolutionised public security, biometric hardware authentication and synthetic media (deepfake technology).