AI Opportunities for the Battery Industry
Understanding a battery management system presents a complex modelling paradigm, mathematically and computationally.
After highlighting the need for better battery management systems as well as the growing demand from customers for accurate predictions, this article presents the current technology limitations, benefits and challenges, and discusses the prospective future for artificial intelligence to be used within the Electrical Vehicle (EV) industry and battery market.
The need for better battery management systems in the battery industry
The energy storage and battery industry is currently going through a technological renaissance as the perturbations of the Electrical Vehicle (EV) sector continue to grow, building increased demand for battery management modelling.
To add gravity, the EV sector today has a concoction of environmental and economic drivers catapulting the market into the forefront of energy storage, providing significant incentives for companies to accelerate the research of battery management systems.
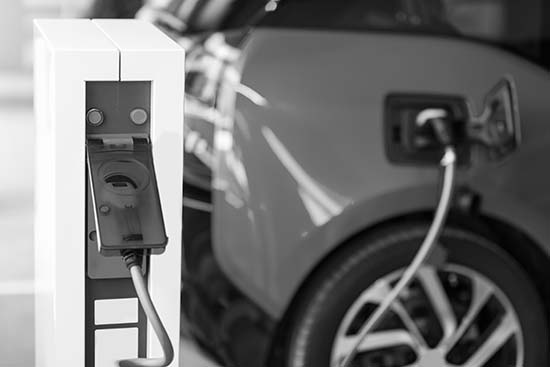
The current evolution of government policies is in favour of constricting CO2 emissions and controlling the collateral damage caused by environmental pollution.
Consequentially there have been strong investments seen in the EV sector with the market's most significant demand for increasing battery pack range, and augmented thermal management cooling systems expected.
Due in addition to these various factors above, the force applied to battery research to increase the accuracy and efficacy of management systems has also grown exponentially.
The current research focuses on Lithium-ion chemistry due to the advantages posed relative to other available chemistries:
- Lithium-ion batteries operate at higher voltages generating high power density.
- Lithium-ion batteries have a low self-discharge rate relative to other chemistries.
- Lithium-ion batteries have a wide operating temperature range whilst also being compact allowing for a longer lifespan.
- Lithium-ion batteries operate using an intercalation system, where the cathode and anode structures allow for reversible insertion and extraction of lithium ions.
- Lithium-ion and lithium-ion cells have high electrochemical potential.
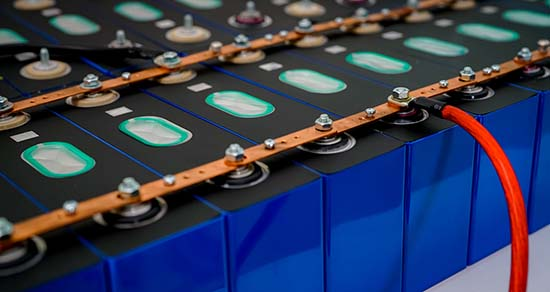
Battery management system current limitations and challenges
However, as it stands, EVs and the current existing chemical and physics-based models of manufacture are confronted with a range of drawbacks low cost, current limits, and design challenges.
Regarding vehicle battery modelling, a range of models exist that are designed to return diagnostics of the battery.
These battery management technologies and diagnostics include predicting, for example, over-voltage, current, battery temperature, overpotential, overcharging, state of charge, state of health, and so forth.
Although these variables are critical to battery longevity, charging, and performance in normal and stressful conditions, the existing models still harbour a degree of inaccuracy due to the nonlinearity of the governing physics and chemistry of battery materials and technologies.
This is especially true for longer-term battery management system predictions, which are expected to clash with the current drive from electric vehicles to increase battery life and reduce them.
There exists a complex and dynamic challenge intertwining electrochemistry and microscale physics to produce accurate battery performance predictions.
The modelling complexity arises due to the technical nature of model parameterization: the model must account for dependencies that exist within the macro and micro scales.
For example, lithium-ion diffusivity and electrical conductivity have a dependence on the concentration of lithium-ion cells. The technical multiscale dependencies weave together a multiplex modelling challenge for battery models.
The infrastructure of battery research is therefore not parsimonious in the number of tests delegated and conducted.
Why is machine learning a good solution for battery management system optimisation?
By compounding the battery model sensitivity and coupled non-linear complexity, there exists a logical drive for a machine learning (ML) approach to battery management system optimisation.
Interchangeable physics and battery modelling libraries present a large amount of existing data for machine learning to digest and optimize.
This battery data is further augmented by the fundamental nature of advanced deep learning techniques enabling the discovery of higher dimensional relationships which are difficult to parameterize.
Machine learning approaches to battery modelling can produce key battery diagnostics discussed above, with relatively high accuracy whilst also computing these predictions at an accelerated rate.
Therefore, ML approaches and AI adoption can provide an enhanced simulation environment for engineers who wish to learn how to conduct experimental tests or evaluate the positive impact of various optimizations; from testing thermal management, battery life, functional safety, and beyond.
AI capabilities have huge implications for the global battery industry market. This technology can also enable battery research users to explore analytical relationships within the battery cell infrastructure (i.e., looking at less charged cells or the most charged cells) which can aid the companies in reducing the time taken for producing optimal-performing battery cells.
How can AI augment battery cells and battery management systems in the battery industry?
Machine learning models thrive with access to large datasets due to the mathematics governing the algorithms. This access, in turn, enables the large datasets gathered through battery management system testing or vehicle drive tests to be effectively integrated.
Real-time energy data that is derived from vehicle driving tests contains information such as the acceleration, ambient temperature, velocity, and state of charge, all of which are a dynamic function of time.
Understanding the relationships that exist quantitively between these variables and each other with time stimulates a need to learn that for AI applications in this industry.
The attraction for AI here exists as it may contribute to understanding complex higher-dimensional relationships which are present between the features, and as a result, leading to a greater understanding of ground truth physics.
Battery testing equipment produces substantial amounts of data that machine learning models can use to decipher relationships.
Understanding the interrelation between the physics defining a battery model allows for intelligent iterations to quickly be made to physical models, which in turn can optimize battery performance.
The current trajectory for the integration of AI and battery physics modelling is to enhance the user's ability to intelligently engineer changes within the battery cell.
The perturbations of intelligent modification and refinement of a battery system are to optimize battery performance and energy storage.
The additional advantage of using AI with physics-based modelling comes from the non-linearity and complexity of the governing chemistry and physics on the atomistic scale.
This high degree of parameterization presents mathematical friction to modelling.
Deep learning techniques therefore can be supplementary in producing digital battery twins sometime in the future.
AI opportunities for the battery industry and battery management system development
More than ever, there is a need for performant and long-lasting batteries, mainly driven by the growth of the worldwide EV industry and their ongoing quest to employ the most effective battery management system in their EV designs.
However, the complexity of the physics of such battery management systems means that battery performance models are not accurate enough, and extensive testing and development are still required to design optimal battery systems.
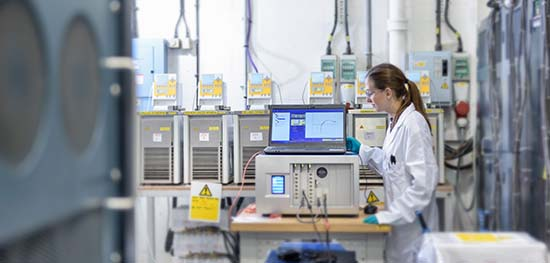
This is where AI can save the day for the battery market, and aid the testing and creation of the optimal battery packs and energy management system for EV's and beyond.
By capturing higher dimensional relationships from battery data gathered from driving tests and other physical tests, AI can better inform battery designers on what their next step should be to design, build and manufacture more robust and performant batteries.
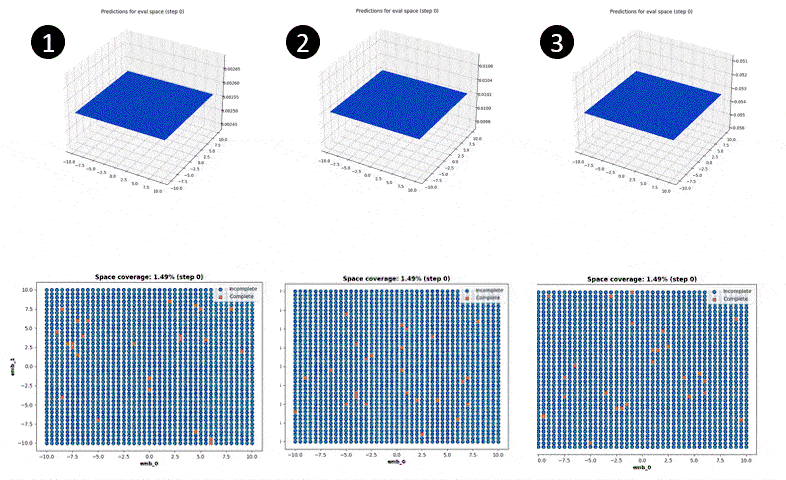
The integration of robust active learning algorithms in our Next Test Recommender (NTR) signifies a significant advancement in product development for safety-critical applications such as battery systems.