Industry
AI Automotive engineering optimisation with Monolith.
Empower your Automotive engineers with artificial intelligence for product development. Spend less time running expensive, repetitive tests and more time learning from test data.
Get in Touch
On-Demand Webinar
Battery testing with AI:
Build a more efficient test plan you can trust
In the first part of the EV webinar series, we reviewed the latest research on using AI models to significantly reduce the testing needed for EV batteries. In this follow-up webinar, we’ll show how to implement these concepts using Monolith software.
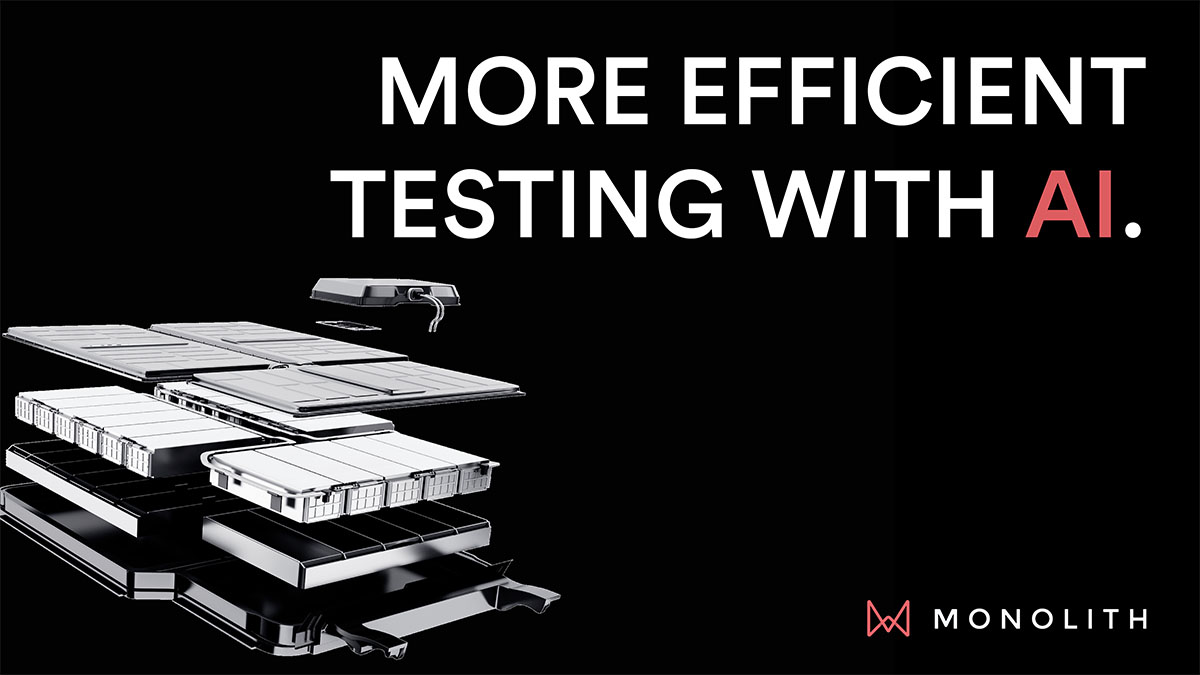
Monolith Software empowers engineering domain experts across all facets of the Automotive industry with AI and machine learning.

Decrease risks to product performance & quality.

Shorten product development duration significantly.
Trusted by leading automotive industry brands:
“With Monolith’s machine learning method, we not only solved the challenge, we also reduced design iteration times and prototyping and testing costs. We are thrilled with the results, and we are confident we have found a way to improve future design solutions.”
-Dr. Bernhardt Lüddecke, Director Validation Global at Kautex
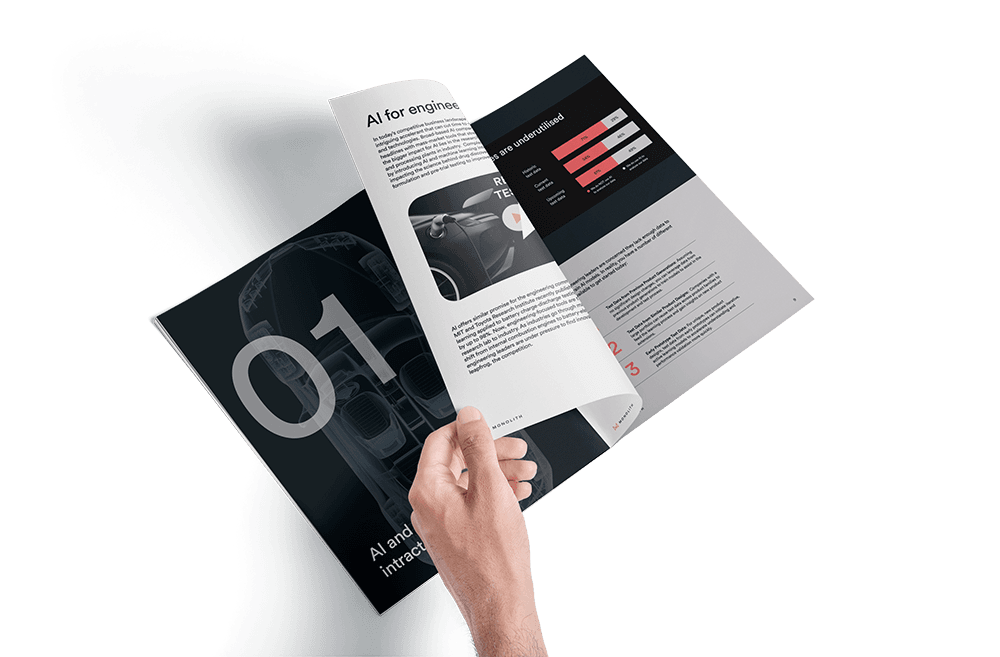
AI for simplifying validation testing
4 applications for AI in validation test
AI has a significant impact on validation testing in engineering product development. You can reduce testing by up to 73% based on battery test research from Stanford, MIT, and Toyota Research Institute. Learn more with Monolith:
Featured Content
Robust active learning for next test recommendations
Integrating Monolith in your verification and validation process can enhance operational efficiency and streamline testing procedures, reducing reliance on excessive physical tests.
How Engineers Use AI to Improve Vehicle Acoustics
Kautex-Textron Case Study
Learn how test engineers at Kautex Textron use self-learning models to more accurately predict intractable fuel sloshing noise faster.
"If your model is in your data, Monolith will find it. Built by engineers for engineers, Monolith helps you make better models faster."
Dr. Ted Duclos, Monolith Advisor and Former CTO at Freudenberg Sealing Technologies
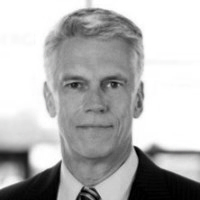
Resources
Discover more AI resources for Automotive
Automotive engineering customers have reported up to a 70% reduction in track testing time, plus a 45% reduction in overall associated costs, while increasing the ROI of costly wind tunnel testing. How can you apply AI to automotive engineering workflows? Get in touch with our team today.