System Calibration Module
Calibrate complex systems faster for key performance requirements
Balance hundreds of input conditions and parameters. Find the best options faster. Move forward with confidence.
Request a Demo
System calibration challenges
Calibrate your system to work properly. Under key conditions. Every time.
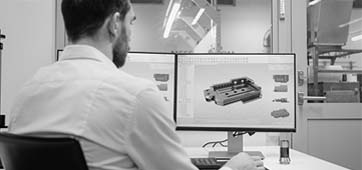
Nonlinear systems are unpredictable
Traditional simulation tools are often unable to accurately reproduce the behaviour and performance of your designs.
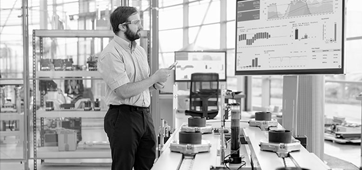
Testing iterations can delay your schedule
Getting the right calibration values requires more testing. Engineers struggle to lock in decisions with confidence.
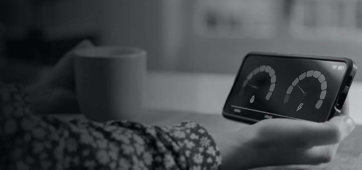
Documentation is required for product certification
Balancing multiple input values for optimal performance across multiple outputs is difficult. Without proof, you can’t ship the product.
System Calibration Module
Calibrate systems with AI-guided optimisation tools.
- Calibrate your system for better performance in less time with less data.
- Make faster decisions with greater confidence.
- Apply tailored test optimisation tools for more efficient data collection required for calibration.
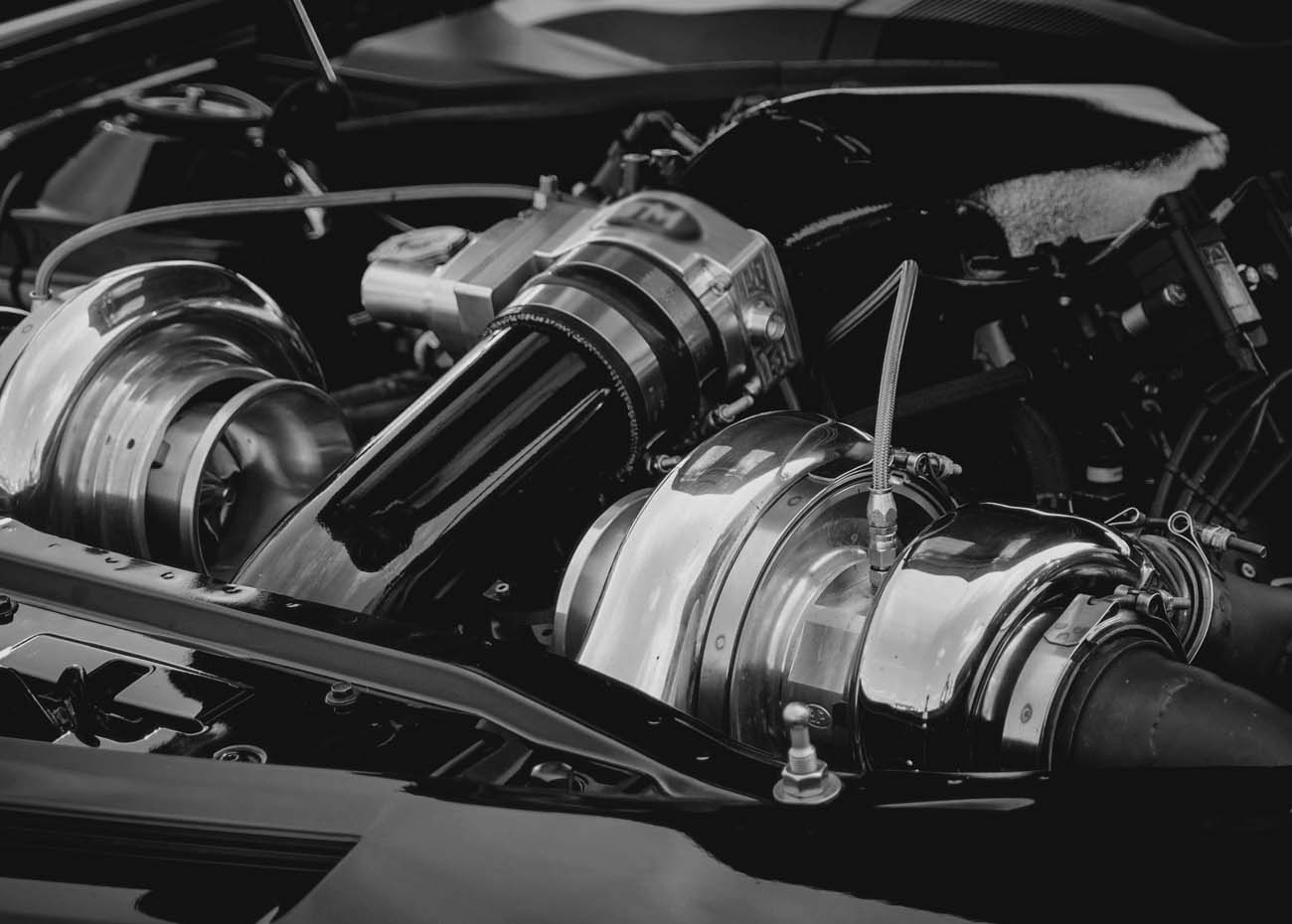
We don’t have the time or budget to continue the level of field and dynamometer testing we're currently doing for calibration data. We need a better way - we think AI can get us there.
-Calibration Engineer, Major US Automotive OEM