Start with a small set of test results or preload your current plan to generate progressive recommendations using AI.
Create more efficient battery test plans you can trust
Reduce test steps by up to 70%. Increase test coverage. Build confidence with an AI-guided test strategy.
Request a Demo
Test validation challenges
A smarter approach for validation testing
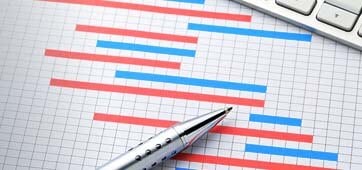
Testing takes too long
Complex products require multiple rounds of prototyping and time-consuming test plans.
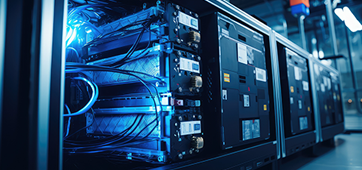
Test systems are expensive
More testing requires more capital costs, higher operational costs, and bigger teams.
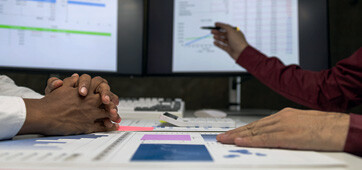
Test planning is difficult
You can never be sure if you’re testing enough (or too much), increasing costs and risks.
Test Plan Optimisation Module
Reduce test plans by up to 70%.
- Run the most important tests and skip the rest.
- Optimise resources spent on costly test rigs.
- Validate your designs faster with fewer prototype iterations.
- Proprietary tool applies thousands of recommender algorithms to model your design space.
- Optimised and refined over multiple years on real customer test plans.
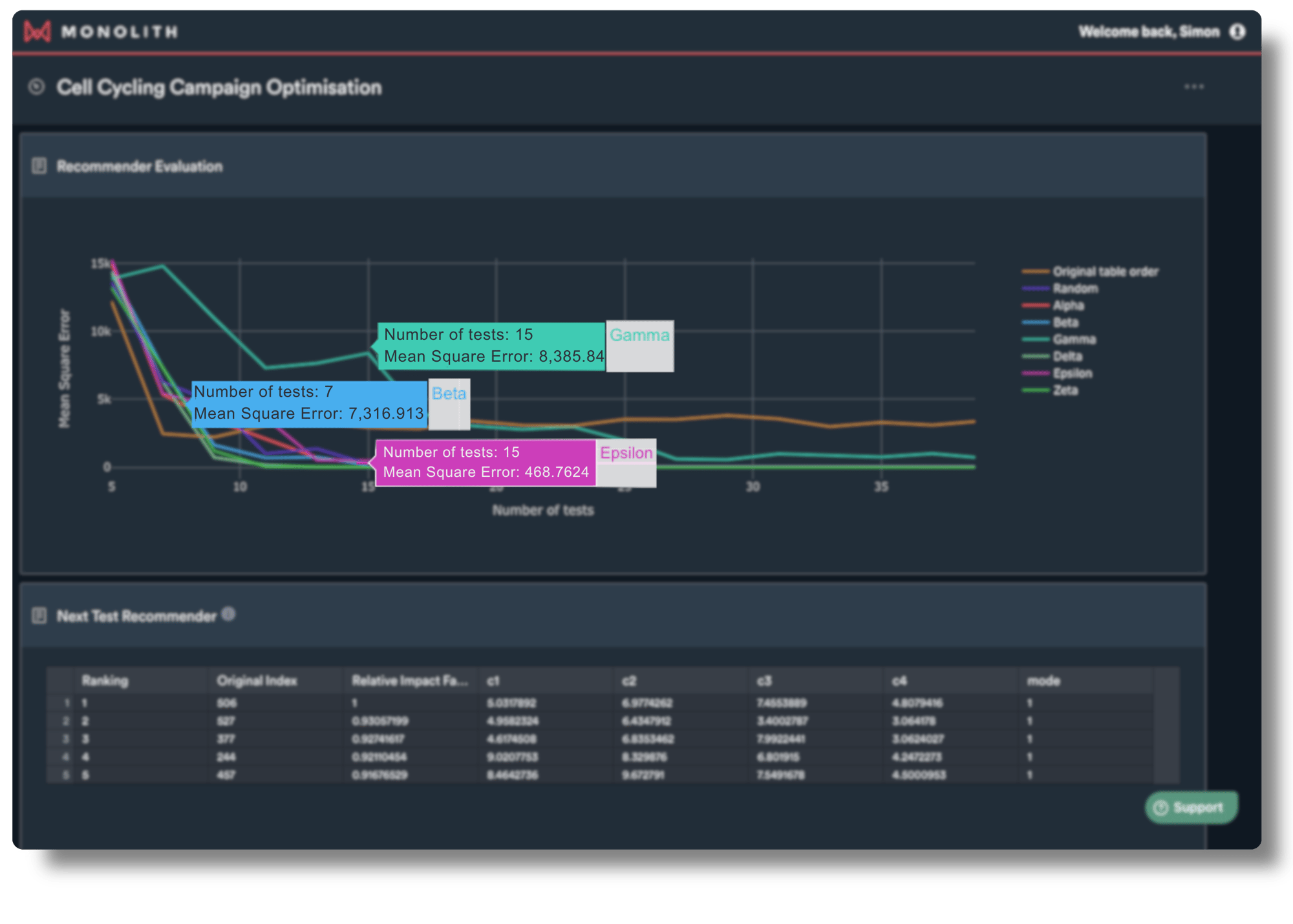
The Monolith team was able to identify faulty assumptions, unnecessary test conditions, and errors in our data using the Test Plan Optimisation Module. With their help, we’ve been able to reduce the number of tests in our plan by more than 70%.
-Director of Test, Major European OEM
How it works
Next Test Recommender
Guide your testing strategy with AI to define a better plan.
Speak to our team
Apply thousands of AI algorithms
Our recommenders apply thousands of algorithms to explore your design space and find the best model.
Generate test recommendations
With each iteration, AI recommenders find test conditions that explore your design most efficiently.
Test with confidence
By optimising your testing plan with Monolith you can reduce the number of tests by up to 70%, and proceed with confidence.
Developing efficient and effective test plans without jeopardising safety and reliability is the top barrier to bringing EV battery solutions to market.
-2024 Forrester Survey of EV Battery Engineering Leaders
Download Forrester study
Monolith resources
Learn more about AI-guided next test recommendations
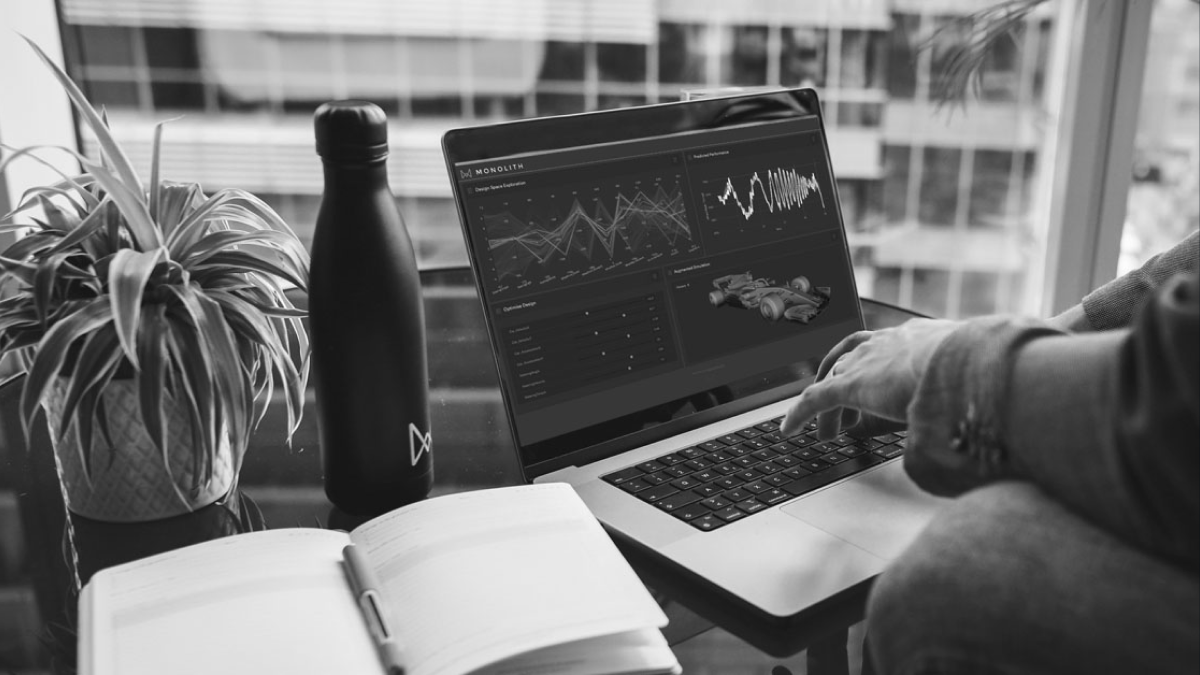