Over the last 20 years, there has been a rapid expansion of systems that use wireless technology, from the growth of the mobile phone market to smart home devices and the continual movement to autonomous vehicles with their smart sensing technology.
Wireless technologies' most important factor: Antenna design
A key enabling component within all of these transmitting devices is the numerous antennas, and respective antenna designs, which are often hidden from human view.
Antenna performance is key to wireless technology operation and efficiency.
I have been fortunate to spend my career working through this technological revolution in particular focussing on the development of the antenna, improved antenna design, and its associated systems.
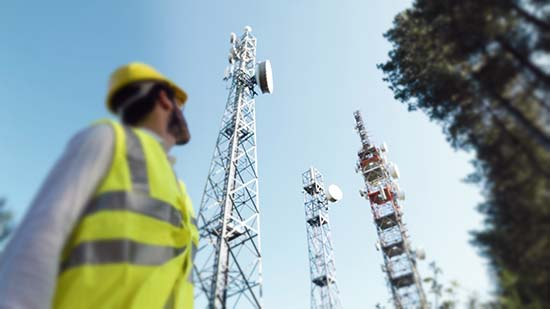
How can I optimise an antenna design?
Recently I had an interesting question posed to me which was, “how do you optimise the antenna?”. This got me, and our team at Monolith AI thinking about the antenna design process.
More specifically, we considered the various tools that we have used over the years, in particular optimisers and optimisation tools to increase efficiency.
As in many areas of engineering, computer simulation is now the mainstay of antenna design (an example of which is shown in Figure 1), with many excellent simulators which allow you to analyse antenna design, radiation pattern, and antenna behaviour in a fully three-dimensional space.
Nearly all of these tools offer a plethora of optimisers that allow you to vary key dimensions (positions, width, spacing, coax cable placement, etc.) and output parameters of the antenna design automatically to meet the required performance.
Antenna design considerations & antenna design parameters
four basic antenna design considerations that should always be addressed are system requirements, antenna placement, antenna selection, antenna element design/simulation, and antenna measurements.
The standard parameters of antennas are bandwidth, antenna radiation pattern, gain, beamwidth, polarization, and impedance.
The antenna pattern is the reaction of the antenna to a ground plane wave incident from a given direction, or the relative power density of the wave transmitted by the respective antenna, in a given calculated direction.
However, in practice one can still find that they need to undertake many of these tasks manually, carrying out small antenna design parameter variations to understand the impacts and trends in performance. So why not use the optimisers?
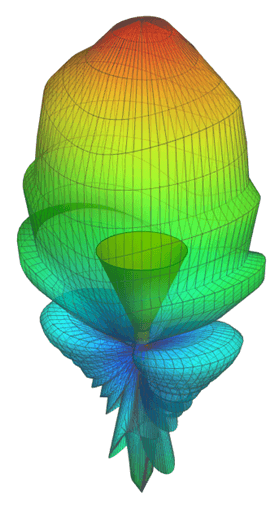
Figure 1: Example of a simulated antenna gain pattern from a horn antenna design.
A lot of this comes down to the antenna behaviour and basis on which many of these optimisation algorithms work. Many tools use mathematical functions which search for maximums or local maximums.
Whilst these do indeed often provide the desired results, the challenge of realising the resulting design can be difficult. Physical manufacturing processes and tolerances are often not well captured with conventional optimisers.
Antenna gain and radiation
Consider for instance Figure 2 below. If we are optimising the length of a structure to give the best antenna gain, the optimiser will give you the “best” result based on finding which length gives you the maximum gain.
Antenna gain is the capacity of the antenna to radiate power more or less in any direction compared to another hypothetical antenna design.
Unfortunately, too often the “best” value is at a sharp point on the curve so any slight variation of length soon leaves you with poor performance (see point 1).
It is often better not to be in the “best” place, but in an area with slightly poorer performance that is still acceptable, but where for example your value of antenna gain varies much more slowly with length (see point 2).
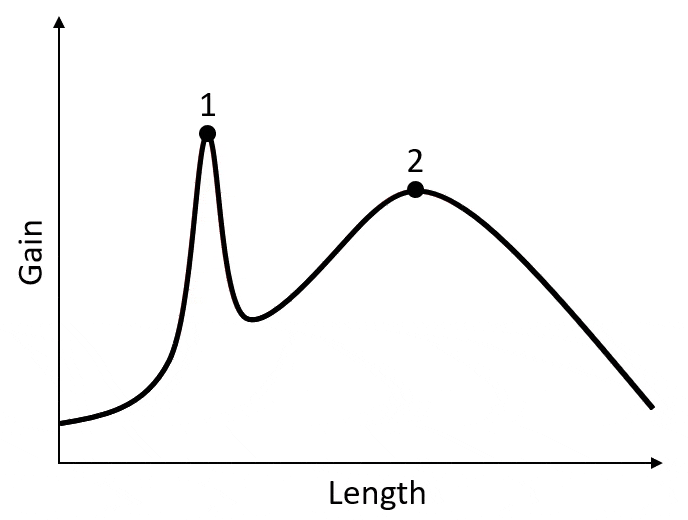
Figure 2: Choice of maximum and tolerance implications.
Another challenge is that you are generally trying to optimise for multiple antenna performance factors, not just say the antenna gain, but also how well power is transferred to and from the electronic circuits it is connected to.
Antenna function and performance factors for antenna design
Often these differing performance factors impose different requirements from the antenna structure, so it always becomes about balance and finding the best compromise.
In my experience, this has been best achieved with a more manual approach, which gives a more in-depth understanding of the design space.
This coupled with feeding in an understanding of the manufacturing processes generally produces a viable design, where some very targeted use of optimisers can be used to further enhance this.
What does this mean for computer optimisation? Well like the technologies we create engineering design processes are also continually evolving and Artificial Intelligence has entered this sphere.
Over the last year, I have been fortunate enough to experiment with this through Monolith AI.
AI adoption for antenna design
Monolith AI is a cloud-based software that enables engineering companies with historical data to explore their data easily and interactively and train machine learning models.
These models are then used to accelerate product development cycles by reducing the number of simulations and tests needed to reach the final design of a product.
My experiments here started with creating a design dataset for a horn antenna.
In my case, this was built theoretically in excel, but in practice, this design set would comprise previous design data from simulations, tests, and possibly some theoretical data.
The beauty of this approach is that inherent in previous design data is a lot of the realistic effects that need to be captured.
For instance, measured results contain all of the impacts of manufacturing imperfections and tolerances.
The Monolith AI software uses this data to train neural networks, which form the basis for growing future designs.
Using my theoretical data set I was interested in designing a horn antenna suitable for feeding a parabolic reflector.
These reflector antennas are seen widely in many applications from home satellite dishes to large radio telescopes.
For best performance, the power from the feed horn should illuminate as much of the dish as possible, whilst not spilling over the edges.
Visualising antenna performance in our AI dashboard
Visualisation of the data set within Monolith, allowed a snapshot of the overall design space to be developed and provided a rapid assessment of the impacts of the different design parameters.
This showed that the radial length (R) of the horn was key (see Figure 3).
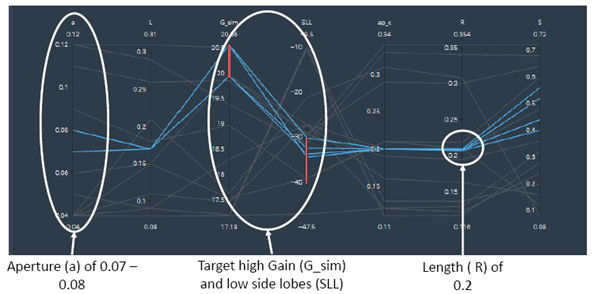
Figure 3: Visualisation of key parameters in horn antenna, blue lines indicate design variants with Gain above 20dBi and sidelobe levels below -30dBi.
Once this was fixed at the optimum level, it was easy to see the relationship with the horn aperture, and to tweak this to maximise the performance (see Figure 4).
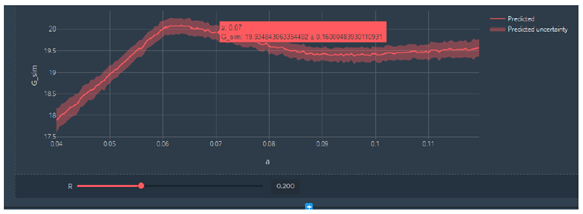
Figure 4: Variation of gain with aperture size for a fixed radial length for antenna design.
It seems that AI is allowing engineering optimisation to enter a new era, one that enhances many of the processes that engineers would have to manually execute during new technology designs.
The ability to visualise and understand multiple design components, parameters, trends, and relationships is powerful, providing the engineer with an immediate understanding of where they are in the design space.
Optimisation based on the whole design space rather than just one or two key components or parameters gets to a realisable design quickly, saving both time and money.
It looks like the type of optimisation tools engineers really require to achieve their goals, such as AI solutions provided by the likes of Monolith AI, are finally here.
AI or machine learning (ML) is an innovative approach to data analysis that automates analytical model construction.
As antennas, and their design process, are becoming increasingly complex, engineers can take advantage of this technology to develop trained models for their physical antenna designs, and execute quick and intelligent optimisations on these models.
Get started with Monolith AI today!