The global market for smart meters, including water, gas, heat, and electricity, is expected to reach $20 billion in 2022.
However, many factors are contributing to the increasing difficulty of building reliable smart meter systems and smart meter technology.
These include an outdated smart meter infrastructure, fast urbanisation coupled with increased energy usage, and rising costs for testing and developing these devices, among others.
Smart meters and smart meter systems must fulfil complex regulations, operate in harsh climate conditions while also reducing non-revenue water losses, as well as fulfilling a carbon-neutral and sustainable future under mounting time-to-market pressure while increasing product performance.
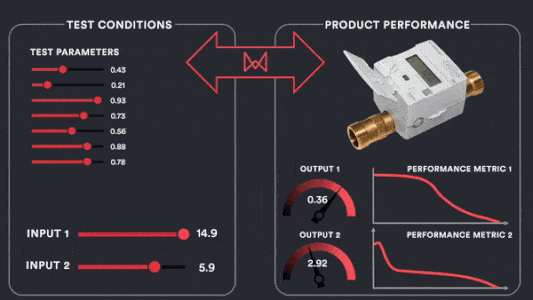
Dragging sliders help engineers to generate new ideas and make quick design choices.
New regulations that define metering accuracy (measuring electricity consumption along with water and gas), fluid flow rates, valves, and advanced metering infrastructure technology require stringent calibration and regulatory tests.
For a test engineer working on smart meters instead of traditional meters, Self-Learning models can be the key to success in leveraging existing and new engineering data from smart meter technology, while not relying on expensive, time-intensive, and repetitive tests.
Ensuring accurate measurement of gas usage has widespread benefits: smart meter engineers can track and predict their own expenditure, while suppliers can understand their client base and provide a more reliable and bespoke service.
Monolith is a no-code application that offers engineers a new way of leveraging their smart meter test data to help them explore, understand and predict performance, thereby reducing test times by up to 70%.
Additionally, Monolith offers collaboration features to ensure institutional smart meter knowledge is capitalised on, shared, and documented for generations to come to innovate new metering devices.
The next generation of smart meters and smart metering technology
When designing the next generation of smart meters for smart grids and beyond, it is important to keep several key smart meter factors in mind.
With higher reliability, utility companies spend less on old meters by maintaining new smart meter infrastructure to ascertain utility energy consumption and reduce overall operating costs (to save money) with each smart meter installed.
Utility companies have proven that smart meters lower energy consumption (increasing energy efficiency and effective power consumption), can detect anomalies, provide cost savings and accurate readings, and lower maintenance costs for utility companies and consumers alike.
Smart metering ensures that smart meters can operate as a real-time data meter reader, and last longer on a single battery while providing two-way communication and giving real-time feedback.
Additionally, with smaller smart meter sizes, more sensors can be placed in more locations further increasing the usage data “intelligence” of how much energy is used and general energy consumption stats (as well as water consumption or water use, natural gas use, etc.), as well as the efficiency of the 'smart grid'—whether water, gas, heat, or electricity.