Start with clean test data to model your system and train the detector algorithm.
Pinpoint battery test errors in seconds
AI-guided anomaly detector finds subtle issues in your measurement data across hundreds of signals in seconds. Eliminate wasted test runs with fast detection.
Request a Demo
Test data challenges
Hidden test errors waste time & money
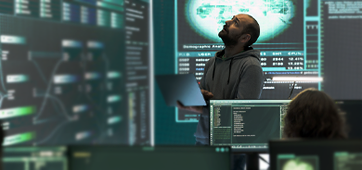
Too much test data
With gigabytes of battery data generated daily, overwhelmed engineers don't have time to validate the results.
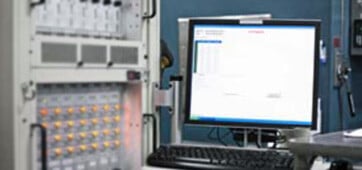
Undetected errors
Wiring and sensor failures, configuration errors, and system defects can go unnoticed for months.
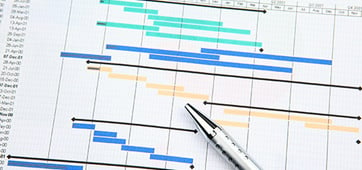
Schedule and budget delays
Measurement issues that linger can mean invalid data, costly retesting, and schedule delays that waste time and money.
Test Data Validation Module
Industry-proven Anomaly Detector finds more errors faster.
- Proprietary algorithm refined with customer test applications and data.
- Find multiple error types across hundreds of channels in seconds.
- Discover issues in individual channels or complex multivariate issues.
- Quickly inspect and prioritise errors using an intuitive data visualiser.
- Validated on sample data to find more than 90% of known errors.
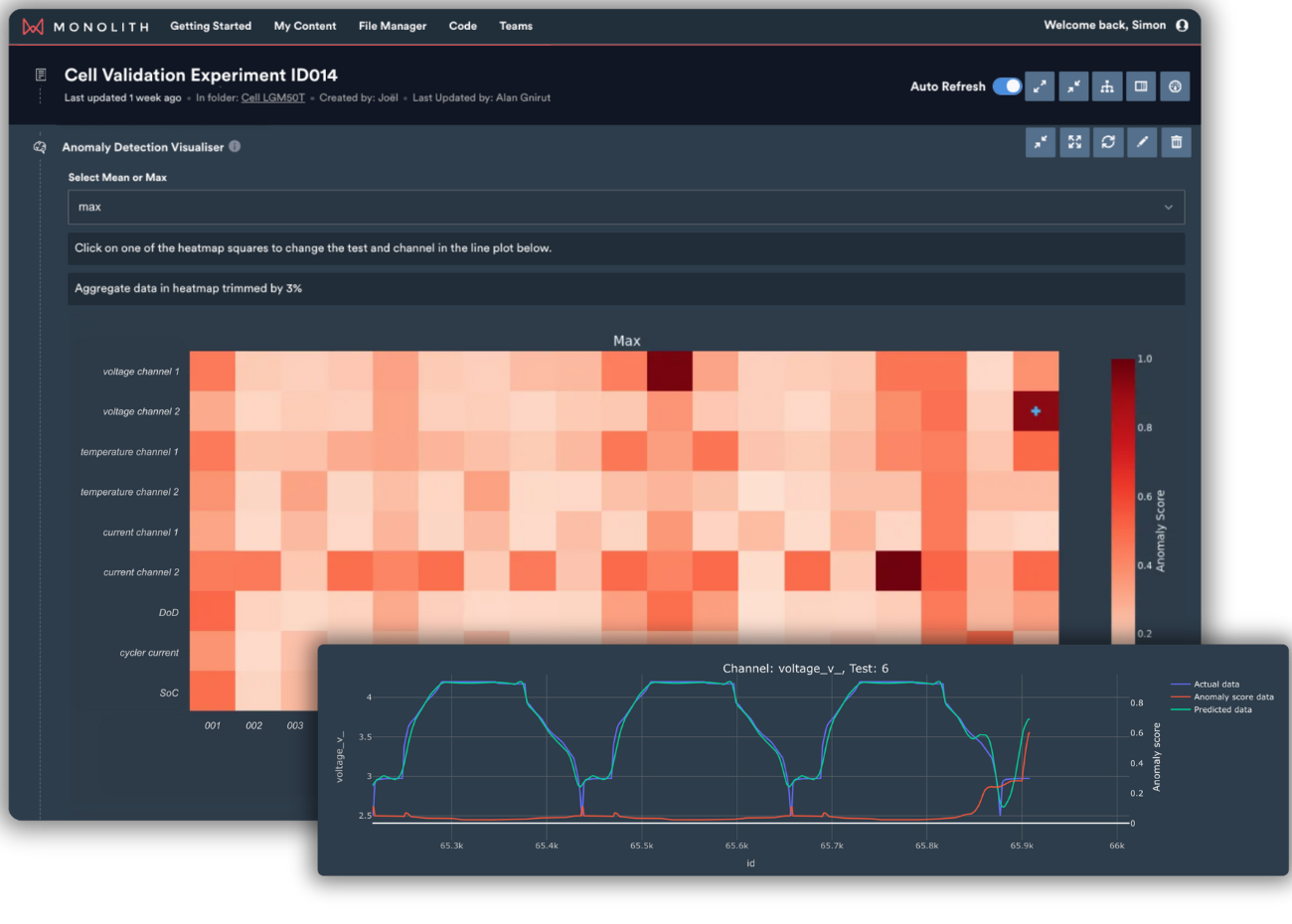
Initial testing showed the Monolith Anomaly Detector found more than 90% of known errors in our test data. We’re able to identify and fix issues in our ageing test results in minutes that previously took weeks to discover.
- Head of Cell Testing, Battery Manufacturer
How it works
AI-guided Anomaly Detector
Speak to our team
Inspect your test data
Apply the detector to your test results to find subtle measurement errors and defects in seconds.
Visualise and prioritise
Explore ranked anomalies with an interactive visualiser to prioritise corrective action.
Automate the process
Integrate with your test systems to automate the detection process to find issues faster.
Monolith resources
Learn more about AI-guided anomaly detection
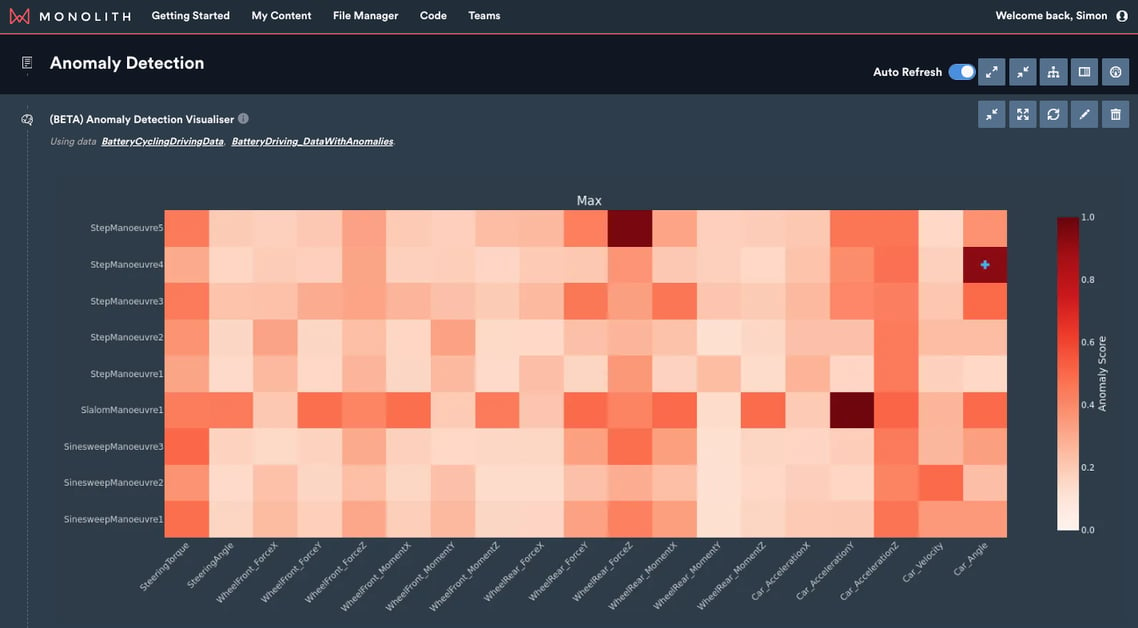