Artificial Intelligence (AI) and Machine Learning (ML) are appearing everywhere across engineering sector industries currently. AI and ML are the shiny, new, advanced-level technological techniques that everyone wants to be using in order to improve time-to-market and speed up product design decision-making ability.
However, adopting any new technology is disruptive to a business, and it can be challenging even to approach. This article will discuss how to progress from the first discovery and trial to deploying an artificial intelligence solution across an entire company in three stages.
Adopting artificial intelligence and affiliated technology for your company should be done in the following three stages:
-
Crawl – prove the concept on one problem.
-
Walk – expand the number of applications and accelerate whole workflows.
-
Run – collaborate with customers and colleagues, and be embedded within the company.
Within these three stages, we'll discuss technical and commercial elements to expect and consider in each stage. As we conclude this six-part series in adopting AI, you will have gained all of our guidance to ensure you are successful in your ambition to get up and running with artificial intelligence informing your business processes.
AI adoption stage 1: starting to crawl
As cliché as it sounds, the adoption of artificial intelligence is a journey. It's a risk that requires an adventurous spirit and the mindset to take on the challenge (see our article about ‘Is your engineering organisation ready for AI?’).
The key is that you need an achievable plan! Before you begin, you need to line up the resources, and the team members and prepare for the effort you will have to put in. The best suggestion is to start small – both figuratively and literally! The purpose of the ‘Crawl’ phase is to prove the concept.
Define a problem to start with
AI cannot solve all our engineering problems. It’s a common AI misconception, that has become even more prominent in the past year. The risk is that in a rush to keep up with advancing technology, you're not applying artificial intelligence to a problem AI can help you solve, which leads to disappointment and frustration in the long run. The key to success is having realistic expectations for AI ability. At Monolith AI, our team works with you to define these expectations and set you up for success.
To start with, identify issues causing you and your business hassle. Then, determine which of these issues can be solved by AI. Within this article, we refer to AI as finding patterns or creating models based on data.
This ability is helpful for engineering problems that are either highly complex or very repetitive. Both types generate the core ingredient for a successful AI problem: data for algorithms to learn from.
Then we need to look more closely at the available data to determine how feasible the problem is to set up:
-
What data is available for that use case?
-
How easy is it to create more?
-
How is the data structured and stored?
-
How frequently is the data produced?
-
What simulation, test, or manufacturing methods generate it?
-
What does the information look like at each stage of the process?
Though a core part of the adoption journey, collecting, cleaning, sorting, and structuring data to apply AI is a daunting task, but we limit the required amount by limiting the scope during this first stage and in the early days.
Reduce your scope
Once you’ve selected your challenge, immediately reduce the scope. I can almost guarantee you’ll have gone too big too soon because everyone gets excited and does! The initial objective for an AI project is to gather enough proof to prove that investing more time and resources will be worth it. You don't want it to be an open-ended exploration.
Set a challenge that you can complete in 1 to 3 months and know how you can measure whether you’ve been successful. Simplify your problem, limit the number of products or the number of test conditions, e.g., testing only one type of yoghurt pot but for a range of different drop tests.
The dataset to describe this should be small to medium in size, with 100s to 1000s of data points. Have five input variables that you want to investigate, and limit yourself to 3 to 5 output variables. Capping the number of variables keeps the problem to a realistic number of dimensions described by the number of available data points. To find out more about what is achievable with different quantities of data, see our article ‘How much data do I need to use AI?’.
It’s a team game
Who is accompanying you on this adventure? It can't be a solo mission to adopt AI, so identify your team. Monolith AI is designed to give engineers the cutting-edge tools that data scientists are developing. Who will benefit most from you resolving this problem? Who understands the dataset you wish to use the best?
Alternatively, do you have knowledgeable data scientists already but no method of disseminating their models into other functions? Finally, your team needs a champion, this is going to take time and effort, and you need an advocate from your organisation who is engaged and can facilitate and unlock access to the data required.
Testing the capability
In this phase, most organisations are looking to test the capability; you are trialling how applying AI can help you solve engineering problems within your business. Can you understand something new about your data? Prove relationships between variables that confirm the existing knowledge in your team? Is it possible to predict an expected result ahead of time? Would it be possible to optimise a design using this model, or is there not enough information yet included?
Learning a new skill
Part of this first stage is also about understanding the strengths and weaknesses of machine learning, so take the opportunity to get familiar with the different algorithms available and when best to use them. Within Monolith, there is a range of models available, and we recommend spending some time testing the different types of machine learning models. See what influence changing model type or model parameters have on the performance.
You can evaluate models for best fit or compare the errors between the model prediction and recorded values. Engineers are naturally curious and need convincing that these models are doing the correct thing and understanding how they work.
Working out where to go next
To progress from the 'Crawl' stage, you should have proved the concept of using artificial intelligence to solve an engineering challenge you and your team are facing. You will have been able to develop in-house data science skills, understand the impact of a variable on performance, speed up the simulation lifecycle or test campaign through prediction, or optimise a new product for release.
At this point, you might be able to visualise the value but will likely not have been able to realise that return just yet (never fear, you're still in the early stages).
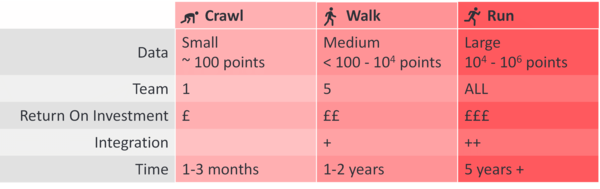
AI adoption stage 2: learning to walk
Now that you’re happy with the principle, you need to ensure that it’s worth your while to continue, and by the end of the ‘Walk’ phase, you want to be generating an actual return on your investment in time and energy! You started small, so now you need to define the strategy for expanding sustainably with artificial intelligence.
Expand your problem scope to other areas
Review the scope of the pathfinders you chose in the previous phase. The models initially built to prove the concept might be suitable for other issues, a concept known as generalising. Or they might need some more information before you can apply them to other problems (more on this is in our article 'creating tranferable AI models for engineering'). Could you add another product to the dataset? Doing so might require including additional features that describe the differences between the current product and the new products. These features describe how to move from one design space to another. E.g., if you had a packaging facility making bottles and your original scope had limited the data to 1L bottles only. You now want to add 2L, 3L and 5L bottles to capture your entire bottle facility. To do so, you are likely to need to add a feature that describes the volume.
Start standardising data collected with artificial intelligence
Think ‘next’; what do you want to develop next? Rather than delving into the last 50 years of data, if you’re fortunate enough to have that history, think about the next design iteration and how it differs from the current data and what features describe those differences and which are common. The recent iterations are likely to be the most helpful and recorded using a similar method, making them less likely to require lots of cleaning and sorting before you can use them.
The standardisation and parameterisation of data is a challenge in itself. For this analysis, you need to have designs recorded in a way that makes them comparable. Expanding your problem scope means bringing in data from other teams about the same design or extending it to other products or factories. This broader data-driven transformation takes time and effort to think about properly. Still, it's worth the investment to be able to reap the value from machine learning across the whole business, and that's where you're heading, so you need to make a start during this phase. (see our article ‘How to share learnings across multiple products using AI?’)
Improve your models with artificial intelligence
Another challenge is that you may have initial models that proved the concept but are not yet accurate for production usage. Use your initial models to identify where the highest errors occur and whether that is due to lack of data in that region, in which case you can see if it's possible to gather more. To control and improve the model, you can also use some AI models' explainability tools to understand your data and find the parameters causing the high variability in that area.
Start using your models for a production application. It might not yet have all the info to be used 100% of the time across your whole design space or product line, but it needs to start working for you in your day-to-day environment so when you next need to analyse a design or set an operating condition, use the model. Now is the time to try it out and make improvements so that the models can be used robustly in the future.
Embed artificial intelligence in your workflow
AI should supplement your existing workflow, set up processing pipelines that enable data communication between your tools and the machine learning algorithms. Data integration is a significant challenge but many tools, including Monolith AI, have APIs to allow the software to draw datasets in and out for real-time analysis.
You can set up templates for appending and cleaning your data. Then frameworks for model evaluation allow the new data created to be added directly into the training set. Then you can monitor how that data influences the model performance and analyse the effect of new data on downstream predictions. These templates should enable data-driven R&D (see our article on How does AI fit into your Engineering workflow?’) and reduce the effort to maintain these models in the future.
Share your journey with others and grow your team
As well as expanding the dataset, you need also to expand your team. As you bring together more of the development cycle data into scope, you'll need more support. Share your progress with your champions and stakeholders. Identify other groups with similar challenges and share your results and what you've learnt with your colleagues. Think both upstream and downstream of where you sit in your company's development process. Can you replicate the project thus far in another area?
At Monolith AI, we recommend increasing the number of applications by two to five more use cases. As the number of artificial intelligence use cases rises, it's essential that the business also acknowledges the additional demand for AI and that you agree on ownership of the solution. Decide whether a central data centre will develop AI, whether it will be led by innovation or embedded within the engineering functions.
By the end of the ‘Walk’ phase, you will have realised the value of your solution and been able to convince others around you of the return on investment of adopting machine learning to accelerate your Research and Development process.
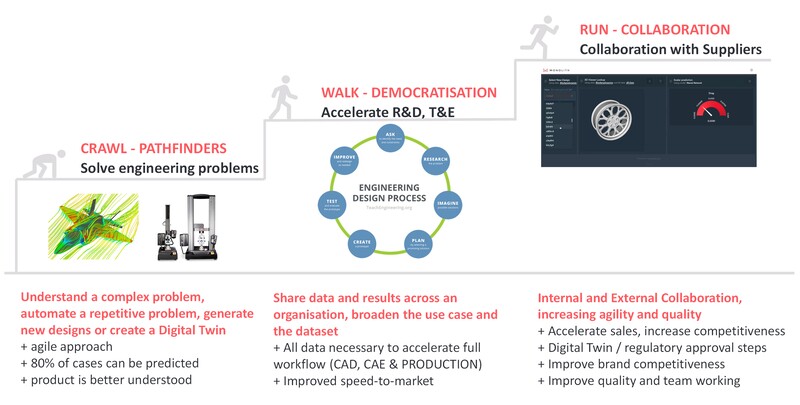
AI adoption stage 3: up and running!
You’re approaching the end of the artificial intelligence adoption journey; this may be several years on from when you set off. The focus now is about controlling the data recording such that it is machine learning friendly – this harks back to our earlier comment about standardisation. Ironic as it may be that to have AI disrupting your development, you need first to standardise it!
Integrate artificial intelligence into the infrastructure
Integration into the existing IT infrastructure is a necessity. This integration will enable you to bring together data from multidisciplinary sources. You can begin to expand the scope of the use-cases you have to bring together data from multiple functions. But these teams typically operate in sequence in a development cycle rather than in parallel, so having the training data for a model that can use data from all these sources is complex.
Tap into the infrastructure for storing incoming data from all the teams. Then ensure that all teams can collaborate on the models and benefit from optimising a process or design with all the parties in mind, considering the performance, cost, manufacturability, recyclability, etc.
Productionise artificial intelligence
Review the models you have built so far; can you deploy any to production? It’s tempting to constantly try to improve the models before trying them in a real-life scenario. Models shared with your internal team need to be continuously maintained, documented, improved, and embedded in the process. Your team will only see a real benefit by interrogating the models in production, understanding their problem in a way they hadn't considered before or, optimising a design without as many iterations. Use the artificial intelligence models and gather feedback to make further improvements.
Bring your customers in on the fun!
Now is the time to share these solutions with your external customers. Many organisations can have a competitive advantage by enabling their customers to interact with an AI prediction of their design performance. Artificial intelligence and correctly employed AI solutions enable almost instant feedback, allowing your organisation to be capable, agile, and competitive in the industry. It’s also an opportunity to recognise your employees or team for taking that initial leap into the world of AI.
There’s no looking back now! We hope you have enjoyed this series on how to get started with your AI journey, and remember that, like most business transformations, it's a marathon, not a sprint.
Break the journey down into smaller milestones, and you'll be surprised at the progress you make! You can speak to us here at Monolith AI, for more research or information from your local guides about the AI adventure, by emailing info@monolithai.com.