Fast-track validation test with AI
Improve product designs, optimise test plans, and resolve product issues faster with AI self-learning models tailored for engineering and test applications.
Request a Demo
AI-Guided Test
Test Less. Learn More.
Monolith unlocks your test data to accelerate product design and validation. Refined from hundreds of AI projects with industry-leading engineering teams, we empower engineers to train machine-learning models to find hidden errors in your data, streamline test plans, find root causes of failures, and build better products - faster.
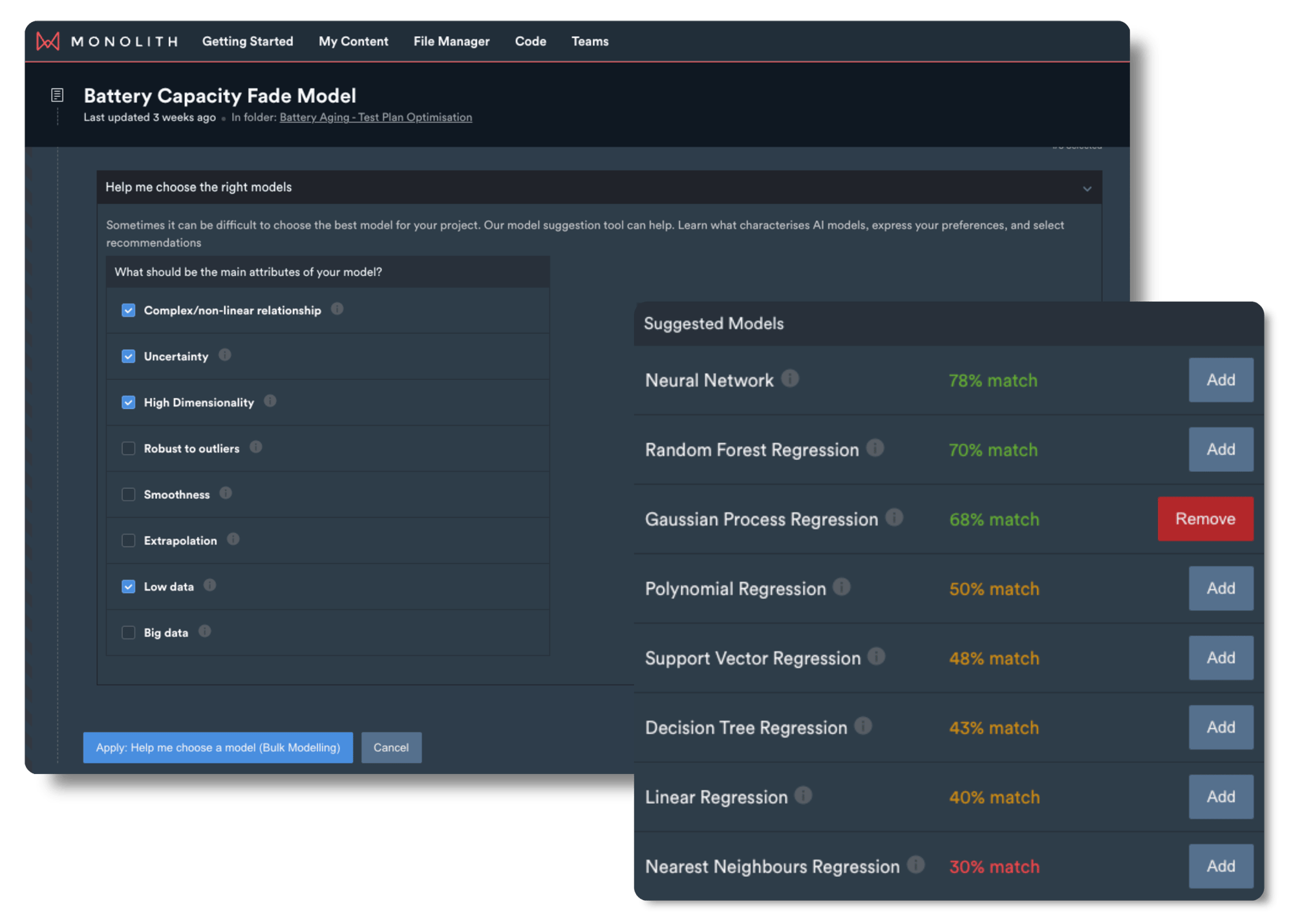
Quickly build interactive notebooks using intuitive dialogs to load, explore, and transform your data for AI.
Train and evaluate self-learning models to quickly find the best fit, with no arduous coding or data science PhD required.
Understand how your product designs work, discover which parameters influence performance, and make predictions for any test condition.
Create a dedicated space for your teams to organise and isolate data, notebooks, and models from others.
Share your insights with your team members or across your department through interactive dashboards.
Rest assured that your data is protected with industry-standard security measures, data encryption, ISO 27001, and access control.
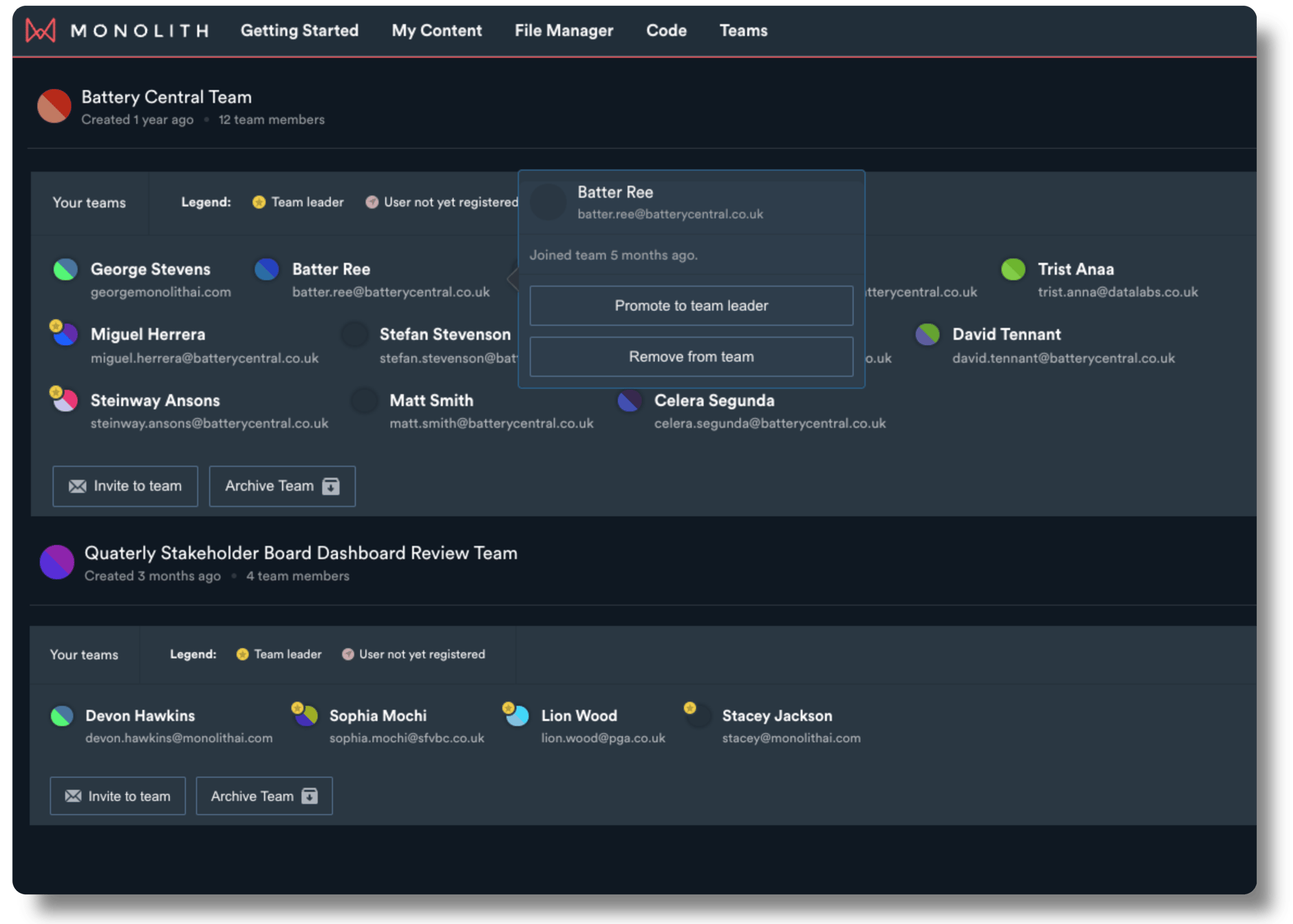
Leverage the cloud for large data sets and advanced computing power to grow as your expertise and requirements grow.
Embed and automate AI models into your engineering processes and tools to realise value and scale from your work.
With multiple configurations and install options, quickly adopt AI without in-house development and infrastructure issues.
Train and upskill your team with in-product tutorials, online learning tools, and custom training options.
Leverage the Monolith tools and expertise from hundreds of AI projects with leading engineering teams worldwide.
You choose the appropriate service level needed to get projects up to speed quickly and ensure long-term adoption and success.
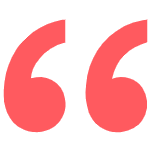
“Initial testing showed the Monolith Anomaly Detector found more than 90% of known errors in our test data. We’re able to identify and fix issues in minutes that previously took weeks to discover.”
- Head of Cell Testing, Battery Manufacturer
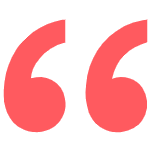
“There are many potential use cases for AI to speed up the battery test and validation process. After an extensive evaluation, we found Monolith to be an excellent option for scaling AI across our R&D.”
- Markus Meiler, VP Research & Development
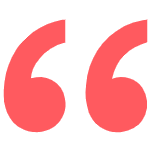
“The Monolith engineering team are unique experts in the field of solving scientific problems with machine learning. I trust them to guide our team to get the greatest ROI from AI and magnify our engineering talent and expertise. ”
- Dr. Paul Gosling, Chief Technology Officer, Thales UK
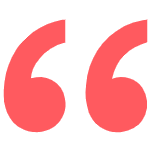
“With Monolith’s machine learning method, we not only solved the challenge, we also reduced design iteration times and prototyping and testing costs. We are thrilled with the results, and we are confident we have found a way to improve future design solutions.”
- Dr. Bernhardt Lüddecke, Director Validation Global Kautex-Textron
Monolith resources
Learn more about AI applications in engineering
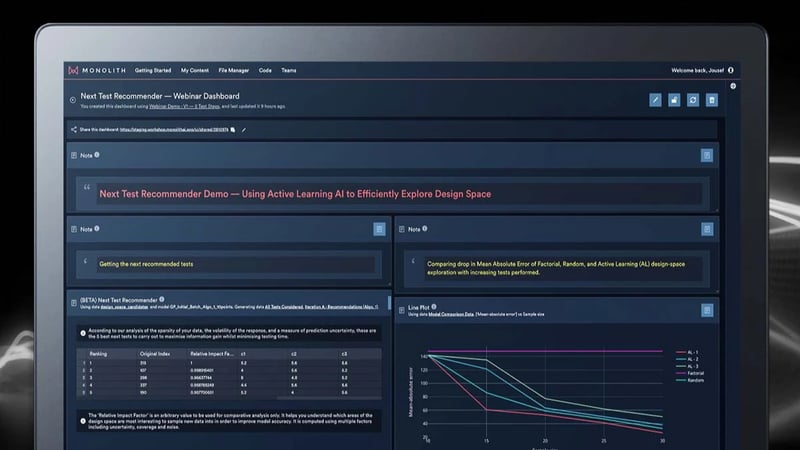