Root Cause Analysis
Find causes for system failures faster
Predict which design changes will most likely fix failures. Avoid long delays and uncertainty during validation.

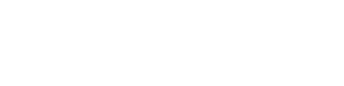
Condition your data to quickly find correlations across your large datasets and disparate sources. Find relationships not readily apparent to the human mind. Respond rapidly to test and system failures.
Product design issues during validation risk launch delays and lost market share. Engineers are under pressure to identify critical parameters causing failure, quickly analyze the root cause, and predict how the product will perform in changing conditions. With Monolith, you can quickly combine and visualize test results from large datasets. Apply tools like resampling and feature extraction to condition the data for better correlations. Use explainable AI tools like sensitivity analysis to understand which inputs are most likely to impact your results to find root-cause and address it quickly.
Find correlations in large datasets quickly

Know more about your design with explainable AI

Respond to failures faster
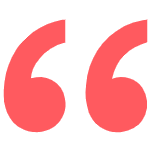
“The Monolith team understood what it means to work with genuine engineering problems in artificial intelligence: the needed flexibility and knowledge.”
- Joachim GUILIE, Curing performance expert at Michelin
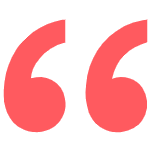
“It's a team with a very good personal relationship.”
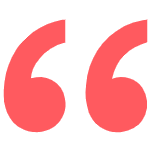
“The Monolith AI team is excellent.”
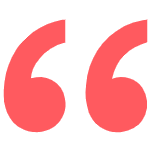
“The best thing about this software is the team. All the team members are experienced and always available to help and solve the issues”
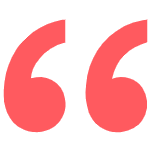
“They are prompt with communication, well organized, really know their stuff, and are good at communicating with non-experts in their field.”
No-Code AI Software
By engineers. For engineers.
- Avoid wasted tests due to faulty data
- Build just the right test plan - no more, no less
- Understand what drives product performance and failure
- Calibrate non-linear systems for any condition
Respond to failures with the power of AI to find solutions faster
Finding the root cause of a system or test error starts with getting all of your data in order. When you are faced with inconsistent results from similar inputs, its time to expand your data set and look for new correlations. Using the data transformation tools in Monolith, you can convert your raw data into features or derivatives to find different relationships or gain more insight into the operation of your design.
Or, you may need to add more data to expand your field of vision. With Monolith's scalable cloud resources, you can add to your dataset and improve your models to learn more about possible reasons for failure.
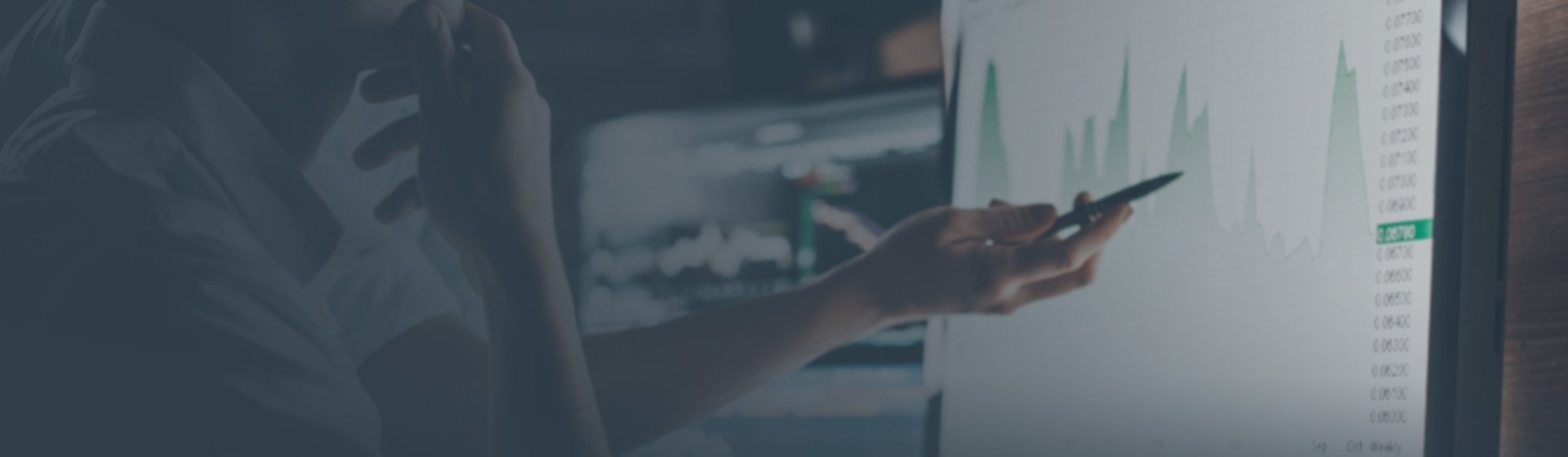
Finding the root cause of a system or test failure starts with knowing the inner workings of your design. Even the most experienced engineers can supplement their inherent understanding of the products they work with AI models to learn more about their design and how it works.
With Monolith, you can build a more in-depth understanding of which inputs and conditions have a greater impact on the performance of your product. Using the advanced visualization tools in Monolith, you can quickly look across many different parameters and see how different values impact your test results to form more intuitive understanding of what is happening and where to prioritize your focus when dealing with failures.
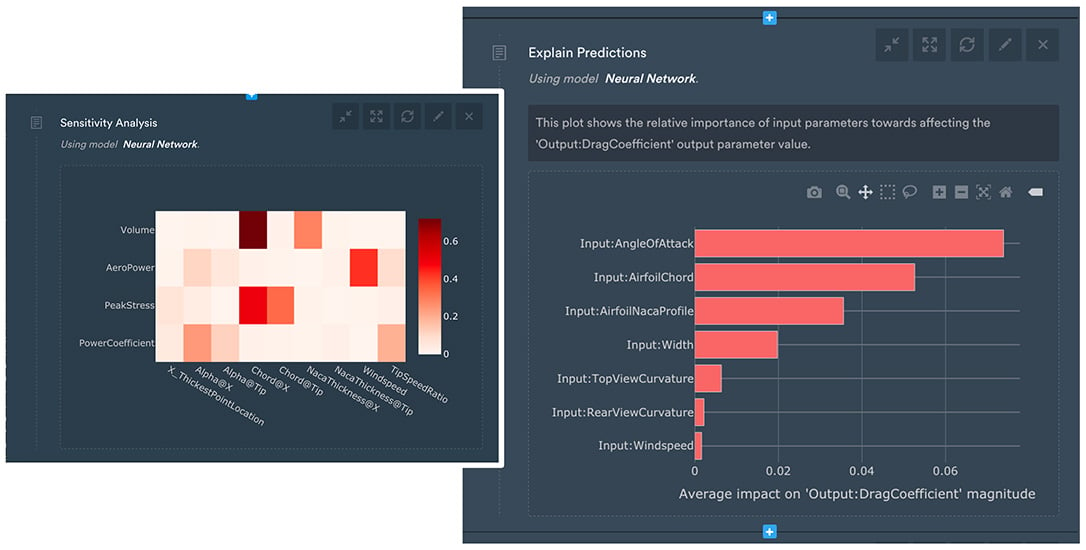
Using advanced time-series auto-encoders, you can reconstruct your design based on test data to make better predictions on how it should perform under different conditions. Using these models, you can monitor test results in near-real time to predict the outcomes and stop tests early if the models predict a failure.
Identifying failures quickly and testing under different conditions help narrow down the options that could be causing issues. You can complex relationships and correlations with machine learning to move with greater agility and confidence when the pressure of failures mount.
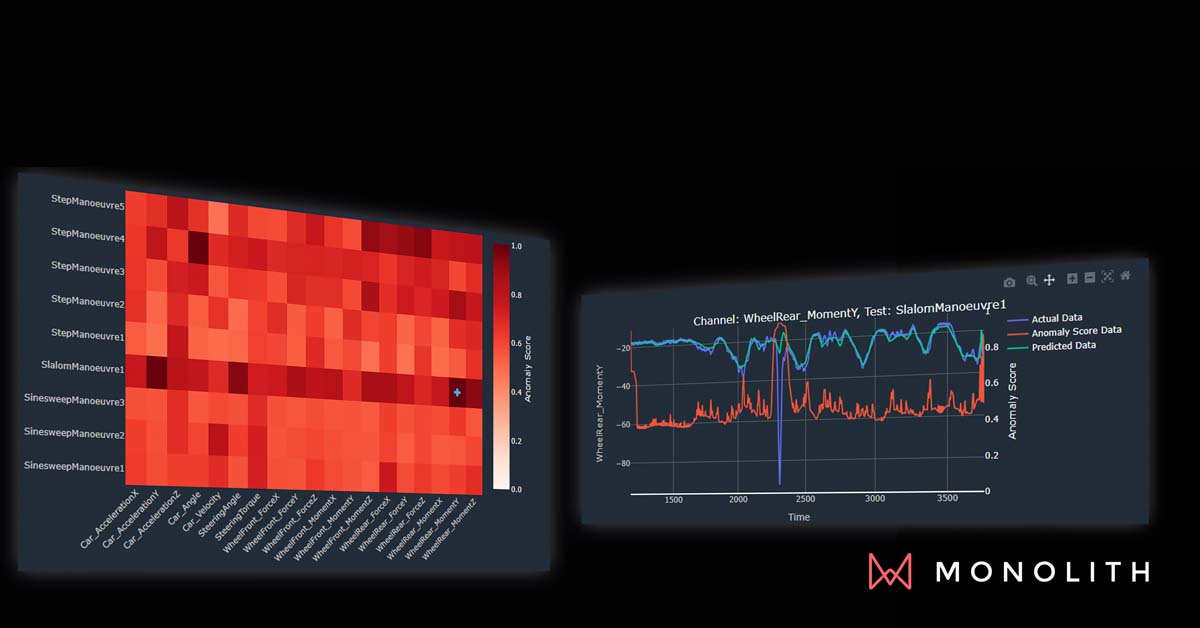
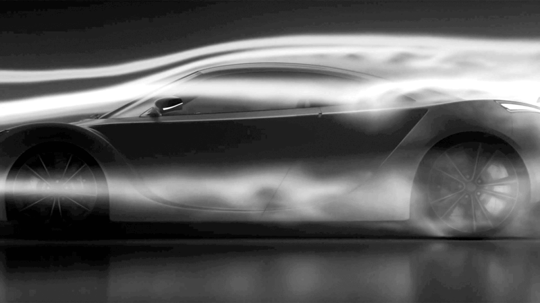
Problem:
Trusting test data
It's vital to understand that testing every possible scenario is not feasible. Over-testing confirms what's already known, while under-testing risks failing certification or missing issues. To optimize testing efforts, identify critical performance components and prioritize tests accordingly.
How We Solve It:
Revolutionised testing
Using self-learning models that get smarter with every test, Monolith identifies the input parameters, conditions, and ranges that most impact product performance so you do less testing, more learning, and get to market faster.
.gif?width=458&height=304&name=ezgif.com-gif-maker%20(17).gif)
Key use cases
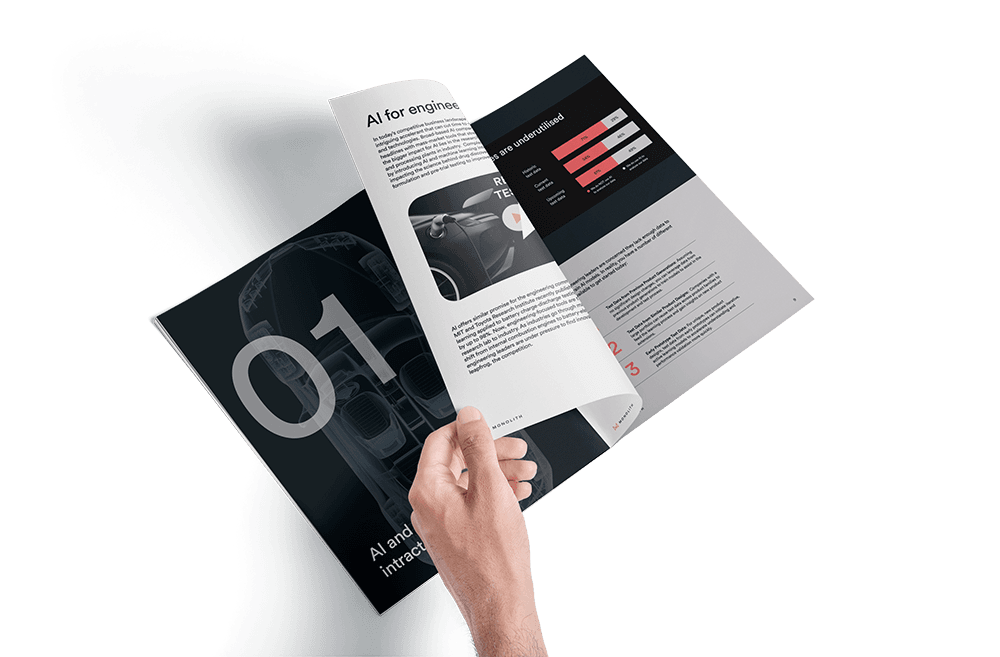
AI for Simplifying Validation Testing
4 applications for AI in validation test
AI has a significant impact on validation testing in engineering product development. You can reduce testing by up to 73% based on battery test research from Stanford, MIT, and Toyota Research Institute. Learn more with Monolith:
Kistler Case Study
72% test reduction using trustworthy AI models
Monolith uses self-learning models to analyse data efficiently, allowing engineers to understand and predict complex system performance quickly. This enhances their workflows and helps solve challenging physics problems. The solution not only predicted vehicle optimisation outcomes but also identified data labeling inconsistencies, leading to significant time savings on the test track.
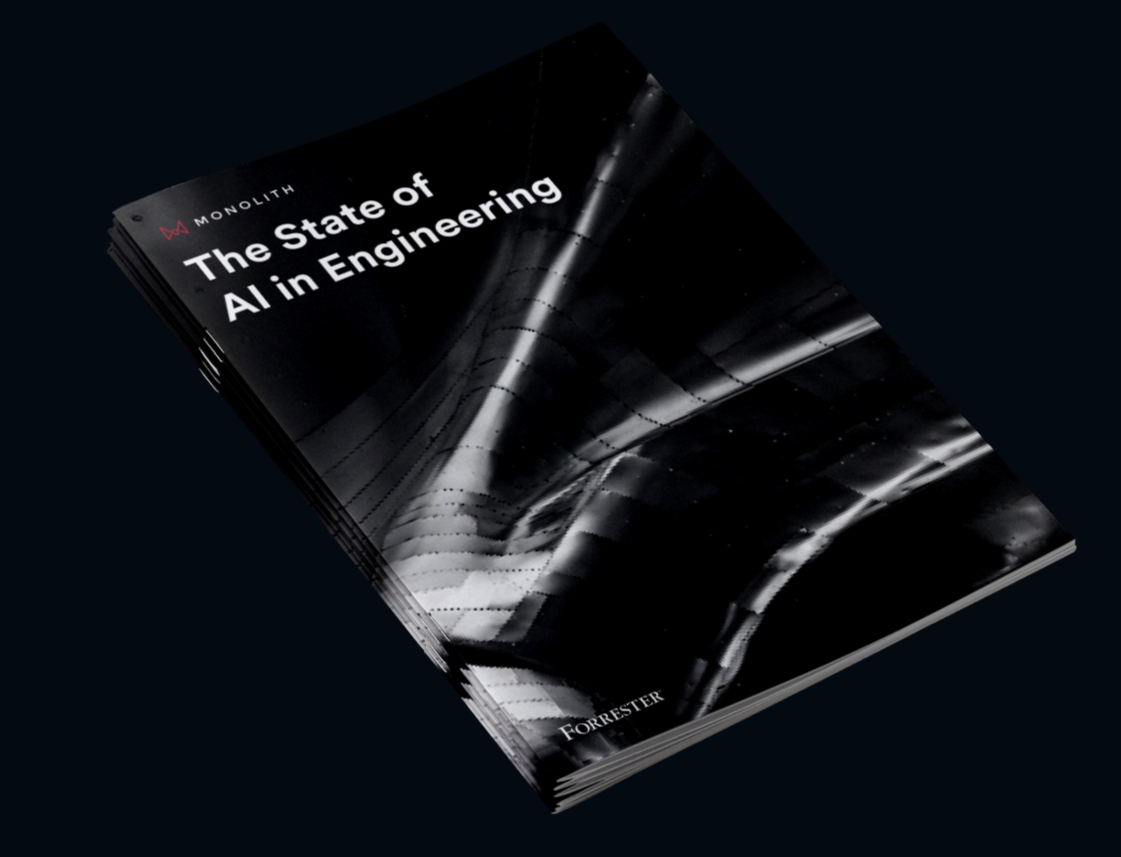
A commissioned study conducted by Forrester Consulting on behalf of Monolith